Linear Regression vs. Logistic Regression: Know the Difference

By Shumaila Saeed & Dua Fatima || Published on July 12, 2024
Linear Regression predicts continuous outcomes, focusing on the relationship between dependent and independent variables. Logistic Regression, in contrast, predicts categorical outcomes, used for classification problems.
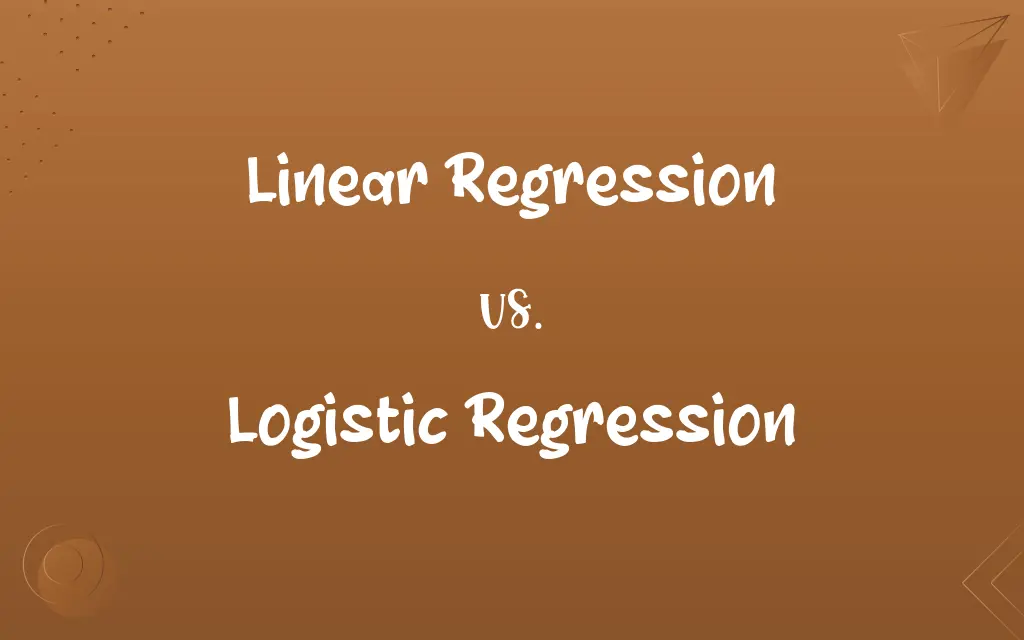
Key Differences
Linear Regression is utilized for predicting a continuous variable, such as house prices, based on one or more predictor variables. It aims to establish a linear relationship between the dependent and independent variables, providing a straight-line prediction model. Logistic Regression, however, is designed for binary or multinomial classification tasks, like spam detection, where the outcome is categorical, not continuous. It estimates probabilities using a logistic function, transforming the linear combination of inputs into a value between 0 and 1.

Dua Fatima
Jul 12, 2024
Linear Regression outputs are unbounded, Logistic Regression outputs are probabilities that are bounded between 0 and 1. This fundamental difference dictates their application areas: Linear Regression for forecasting values and Logistic Regression for determining the likelihood of belonging to a class.

Dua Fatima
Jul 12, 2024
Error measurement in Linear Regression is typically done through methods like Mean Squared Error (MSE), focusing on the difference between the actual and predicted values. Logistic Regression, on the other hand, uses metrics like Log Loss to measure the performance of a classification model, focusing on the probability error.

Shumaila Saeed
Jul 12, 2024
Linear Regression assumptions include linearity, homoscedasticity, and normality of residuals, requiring a linear relationship between the independent and dependent variables. Logistic Regression does not assume a linear relationship between the independent variables and the logit of the dependent variable but still requires the independent variables to be linearly related to the log odds.

Shumaila Saeed
Jul 12, 2024
Linear Regression is sensitive to outliers, which can significantly affect the regression line and subsequently the forecasted values. Logistic Regression is generally more robust to outliers, as it predicts probabilities; outliers have less impact on the classification boundaries.

Shumaila Saeed
Jul 12, 2024
ADVERTISEMENT
Comparison Chart
Assumptions
Linearity, homoscedasticity, normality of residuals
Linearity in log odds, no multicollinearity

Shumaila Saeed
Jul 12, 2024
ADVERTISEMENT
Linear Regression and Logistic Regression Definitions
Linear Regression
Predicts a continuous outcome based on independent variables.
Predicting house prices based on square footage and location.

Dua Fatima
Feb 26, 2024
Logistic Regression
Used for binary classification problems.
Determining if an email is spam or not spam.

Dua Fatima
Feb 26, 2024
Linear Regression
Can be extended to multiple regression for multiple independent variables.
Using both age and weight to predict blood pressure.

Hifza Nasir
Feb 26, 2024
Logistic Regression
Outputs probabilities of class memberships.
Predicting the probability that a tumor is malignant or benign.

Shumaila Saeed
Feb 26, 2024
Linear Regression
Estimates the relationship between variables using a straight line.
A linear regression model may show that salary increases with years of experience.

Shumaila Saeed
Feb 26, 2024
ADVERTISEMENT
Logistic Regression
Does not require a linear relationship between dependent and independent variables.
Can predict outcomes based on categorical data, like drug effectiveness.

Dua Fatima
Feb 26, 2024
Linear Regression
Requires assumptions about the distribution of variables.
Assumes that residuals are normally distributed for accurate predictions.

Dua Fatima
Feb 26, 2024
Logistic Regression
More robust to outliers in classification tasks.
Outlier observations have less influence on determining the decision boundary.

Hifza Nasir
Feb 26, 2024
Linear Regression
Sensitive to outliers, which can skew predictions.
A very large house sale price can distort the regression line.

Shumaila Saeed
Feb 26, 2024
Logistic Regression
Uses the logistic function to model data.
Logistic regression may estimate the likelihood of a student passing based on hours studied.

Shumaila Saeed
Feb 26, 2024
Repeatedly Asked Queries
Why is the logistic function important in Logistic Regression?
The logistic function transforms the linear equation to output probabilities, ensuring the output values fall between 0 and 1.

Dua Fatima
Jul 12, 2024
Can Linear Regression be used for classification?
While theoretically possible by setting thresholds, it's not ideal due to its continuous nature and sensitivity to outliers. Logistic Regression is preferred for classification.

Shumaila Saeed
Jul 12, 2024
Can you use Linear Regression for time series analysis?
Yes, but it's limited to linear trends and often requires additional techniques to handle autocorrelation and non-stationarity.

Shumaila Saeed
Jul 12, 2024
What makes Logistic Regression robust to outliers?
Its classification decision is based on probabilities and thresholds, making it less sensitive to extreme values compared to Linear Regression's continuous output.

Shumaila Saeed
Jul 12, 2024
Is it necessary for the dependent variable in Linear Regression to be normally distributed?
The normality assumption applies to the residuals of the model, not the dependent variable itself.

Shumaila Saeed
Jul 12, 2024
What are the limitations of Linear Regression?
It cannot handle non-linear relationships without transformations and is sensitive to outliers and multicollinearity.

Shumaila Saeed
Jul 12, 2024
What is the main difference between Linear and Logistic Regression?
Linear Regression is used for predicting continuous outcomes, while Logistic Regression is used for predicting categorical outcomes.

Dua Fatima
Jul 12, 2024
How does Logistic Regression handle more than two categories?
It uses multinomial Logistic Regression or extends to a One-vs-Rest approach to accommodate multiple classes.

Shumaila Saeed
Jul 12, 2024
How does Linear Regression deal with categorical variables?
Categorical variables can be included in a Linear Regression model through dummy coding, allowing the model to incorporate their effects.

Hifza Nasir
Jul 12, 2024
How do you interpret the coefficients in Logistic Regression?
Coefficients represent the change in the log odds of the dependent variable for a one-unit change in the predictor.

Shumaila Saeed
Jul 12, 2024
What happens if Linear Regression assumptions are violated?
Violating assumptions can lead to inaccurate estimates and predictions, making model validation and assumption testing critical.

Hifza Nasir
Jul 12, 2024
Why is multicollinearity a concern in Logistic Regression?
Multicollinearity among predictors can obscure the significance of independent variables, making it hard to discern their effects.

Dua Fatima
Jul 12, 2024
Can Logistic Regression be used for non-linear relationships?
Yes, through feature engineering or polynomial regression, non-linear relationships can be modeled.

Dua Fatima
Jul 12, 2024
Can the outcome of Logistic Regression be greater than 1?
No, the logistic function ensures the outcome is always between 0 and 1, representing probabilities.

Hifza Nasir
Jul 12, 2024
How do you choose between Linear and Logistic Regression?
The choice depends on the nature of the dependent variable: use Linear Regression for continuous outcomes and Logistic Regression for categorical outcomes.

Dua Fatima
Jul 12, 2024
Share this page
Link for your blog / website
HTML
Link to share via messenger
About Author
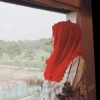
Written by
Shumaila SaeedShumaila Saeed, an expert content creator with 6 years of experience, specializes in distilling complex topics into easily digestible comparisons, shining a light on the nuances that both inform and educate readers with clarity and accuracy.
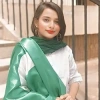
Co-written by
Dua Fatima